The Role of AI in Enhancing Customer Data Analysis
Welcome to the world of advanced customer data analysis, where artificial intelligence (AI) is pivotal in revolutionizing how businesses understand and utilize customer feedback data for optimal decision-making.
With the help of AI, companies can automate and analyze vast amounts of customer feedback data, resulting in improved efficiency, accuracy, and consistency in their data analysis processes.
Best Recommended and Proven Way to Make Money Online – Click HERE for Instant ACCESS >>
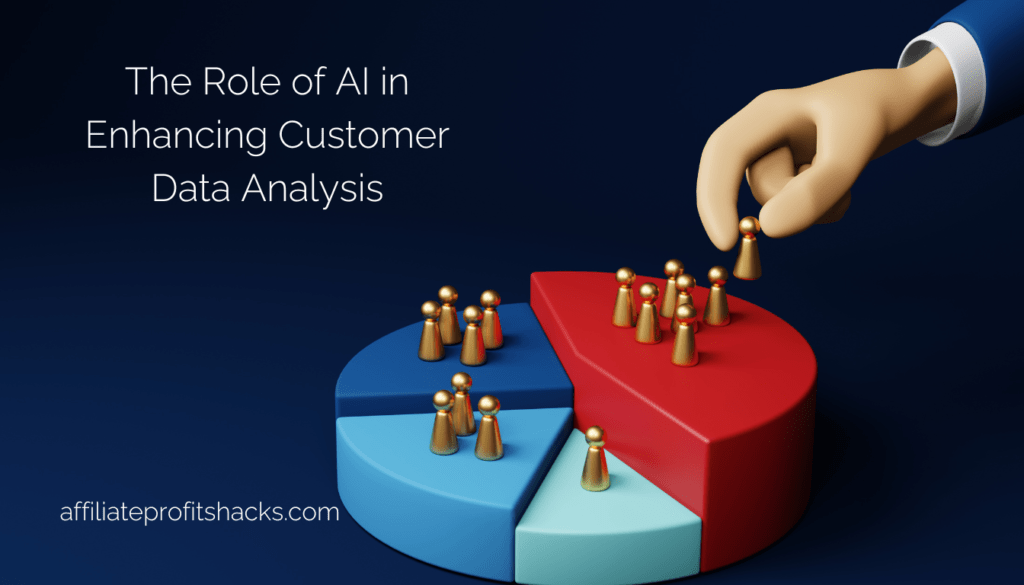
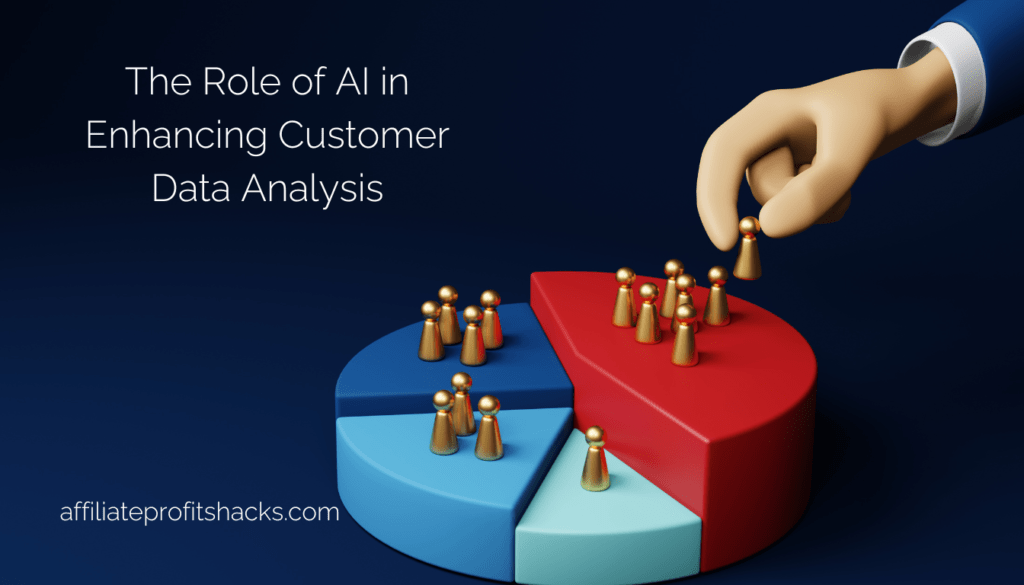
AI solutions for customer data analysis are designed to efficiently categorize and analyze customer feedback data, providing valuable insights into customer behavior patterns, needs, expectations, preferences, and pain points. By harnessing the power of AI, companies can make data-driven decisions, enhance the overall customer experience, and drive business growth.
Let’s explore the benefits of AI in customer data analysis and discover real-life examples of successful businesses that have utilized AI-powered tools to personalize customer interactions, improve products/services, and boost customer loyalty.
Through this article, we will delve into the various aspects of AI in customer data analysis, including enhancing customer experience via AI, advantages of AI in customer feedback analysis, comparing NPS with AI tools, successful implementation of AI in businesses, challenges and limitations of AI in customer feedback analysis, implementing AI in Customer Relationship Management (CRM), and the future of AI in predictive analytics.
Enhancing Customer Experience via AI
Artificial Intelligence (AI) has revolutionized how companies enhance customer experience by leveraging customer feedback data. Through AI tools, businesses can automate and analyze large volumes of customer feedback, uncovering valuable insights that lead to improved products, services, and overall customer satisfaction.
By implementing AI solutions for customer experience, companies gain access to a wealth of information about customer behavior patterns, needs, expectations, preferences, and pain points. This deeper understanding enables businesses to make data-driven decisions that directly address customer needs, enhancing customer experience.
One of the significant benefits of AI-driven customer experience is the ability to personalize communication, marketing, loyalty programs, and inventory management. Companies like Starbucks, with their Deep Brew platform, utilize AI algorithms to provide personalized recommendations and promotions to individual customers. Similarly, Netflix uses AI to deliver tailored content recommendations based on user preferences, significantly improving user engagement and satisfaction.
By harnessing the power of AI, businesses can leverage customer feedback data to improve their offerings and continuously adapt to evolving customer needs. The benefits of incorporating AI into customer experience strategies are undeniable, allowing companies to remain competitive in today’s rapidly changing marketplace.
Advantages of AI in Customer Feedback Analysis
AI in customer feedback analysis offers several benefits that can significantly improve your business operations and customer satisfaction. By leveraging AI tools for customer feedback analysis, you can optimize your data processing, gain valuable insights, and make data-driven decisions that result in better customer experiences.
Efficient Analysis and Automation
AI tools enhance the efficiency of customer feedback analysis by automating the categorization and analysis of large volumes of feedback data. This automation reduces manual work and processing time, allowing you to focus on extracting valuable insights from the data. By saving time and resources, AI-driven customer feedback analysis can significantly improve the overall efficiency of your feedback analysis process.
Improved Accuracy and Consistency
One of the key advantages of using AI in customer feedback analysis is its ability to minimize human errors and oversights. AI algorithms are designed to make accurate judgments and deliver precise analytics, ensuring the reliability and consistency of your data processing and results. This allows you to study growth and behavioral changes over time, leading to more informed decision-making.
Detailed Customer Insights for Better Decision-Making
AI tools provide detailed insights into customer buying patterns, preferences, opinions, and thought processes. By analyzing customer feedback data, AI-driven analysis allows you to uncover valuable information to help improve your products/services and personalize customer interactions. These insights enable you to make data-driven decisions that align with customer needs and expectations, ultimately leading to better customer experiences.
Image for illustrative purposes:
By leveraging the advantages of AI in customer feedback analysis, you can enhance the efficiency and accuracy of your data analysis, gain valuable customer insights, and make informed decisions that result in better customer experiences. Stay ahead of the competition by integrating AI tools into your customer feedback analysis process and unlock the full potential of your data.
NPS Versus AI Tools
Regarding analyzing customer feedback, two powerful approaches stand out: NPS (Net Promoter Score) and AI tools. While NPS is a widely used customer experience metric, AI tools offer a more comprehensive and advanced way to understand customer satisfaction and loyalty.
NPS provides valuable insights into overall customer sentiment and loyalty by asking a simple question: “On a scale of 0 to 10, how likely are you to recommend our product/service to a friend?” However, AI tools take customer feedback analysis to the next level by leveraging advanced technologies like natural language processing (NLP) and machine learning.
AI tools, such as sentiment analysis using NLP techniques, go beyond NPS scores and delve into customers’ emotions, opinions, and perspectives. AI tools can accurately identify the factors influencing customer satisfaction or dissatisfaction by analyzing the language used in customer feedback.
Furthermore, AI tools can analyze large volumes of customer feedback data, identify patterns, and make predictive analyses. They can uncover specific characteristics or demographics contributing to customer satisfaction, helping businesses tailor their products/services to specific customer segments.
Best Recommended and Proven Way to Make Money Online – Click HERE for Instant ACCESS >>
Unlike NPS, which focuses on a single metric, AI tools provide a holistic view of customer feedback analysis. They offer insights into overall customer sentiment and the underlying reasons driving that sentiment. This enables businesses to make data-driven decisions and take targeted actions to improve their products/services and retain customers.
In conclusion, while NPS is a valuable customer experience metric, AI tools provide a more comprehensive and advanced approach to analyzing customer feedback. With their capabilities in sentiment analysis, predictive analysis, and specific customer insights, AI tools empower businesses to gain a deeper understanding and make more informed decisions to enhance customer satisfaction and loyalty.
Successful Businesses Using AI-Powered Tools
Several successful businesses have already implemented AI-powered tools in customer data analysis to enhance their products/services and improve the customer experience.
Starbucks, a well-known global coffee chain, leverages the power of AI with its Deep Brew predictive analytics and machine learning tool. This cutting-edge technology enables personalized communications, targeted marketing strategies, individualized loyalty programs, and optimized inventory management. By analyzing customer behavior and preferences, Starbucks improves its offerings and creates more delightful customer experiences.
The popular streaming service Netflix utilizes AI and machine learning algorithms to suggest personalized content to its users. By analyzing user preferences, viewing history, and other relevant data points, Netflix enhances user engagement and maximizes revenue generation. The AI-powered content recommendations ensure that users are presented with content they are likely to enjoy, leading to increased customer satisfaction and retention.
Another example of AI implementation in customer analysis is the generative AI chatbot developed by OpenAI. This chatbot revolutionizes the customer experience by simplifying customer feedback analysis. Through interactive chat sessions, it identifies areas for improvement and generates new product ideas based on customer input. This AI-driven approach enables companies to prioritize customer needs and continuously enhance their products/services.
These real-life examples demonstrate the benefits and effectiveness of AI-powered tools in customer data analysis across various industries.
Gathering Customer Feedback with AI
AI tools provide efficient methods for gathering customer feedback. Surveys and automated forms powered by AI allow companies to create interactive feedback surveys and collect specific feedback focused on selected products or demographics. These AI-powered surveys can analyze the sentiment and intentions behind customer feedback, providing valuable insights that help companies enhance the quality of their products/services.
Chatbots integrated into apps or websites allow for real-time interactions with customers, enabling quick problem-solving, answering queries, and gathering instant feedback. Companies can improve response times and ensure a seamless customer experience by utilizing AI-powered chatbots for customer feedback.
Social media monitoring using AI integration enables businesses to gather information about product mentions, positive/negative chatter about the business, and market trends. This AI-driven customer feedback collection further enhances customer feedback analysis, providing a comprehensive view of customer sentiments and preferences.
The Challenges of Using AI in Customer Feedback Analysis
While AI offers numerous benefits in customer feedback analysis, it comes with challenges and limitations that businesses need to overcome. One of the main challenges is the presence of intrinsic biases that may affect the accuracy of automated responses. Despite AI algorithms’ advanced text classification capabilities, biased outcomes are still risky.
Another challenge is the need for human judgment in strategic decision-making and interpreting sentiments in full context. AI can provide valuable insights and automate specific processes, but the human element remains essential for complex analysis and understanding the nuances of customer feedback.
The optimal approach to customer feedback analysis is to merge machine learning with human intelligence. By combining the speed and efficiency of AI with human ingenuity, businesses can ensure accurate and reliable analysis. This hybrid approach leverages the capabilities of AI to process massive volumes of data while incorporating human judgment for more nuanced interpretations.
Additionally, businesses must address data privacy and security concerns when implementing AI systems for customer feedback analysis. It is crucial to comply with data protection regulations and prioritize the ethical handling of customer data to build trust and maintain customer transparency.
Overcoming the challenges of using AI in customer feedback analysis requires a careful balance between AI-driven automation and human expertise. By embracing the strengths of AI while mitigating its limitations, businesses can unlock the full potential of customer feedback analysis and make more informed decisions.
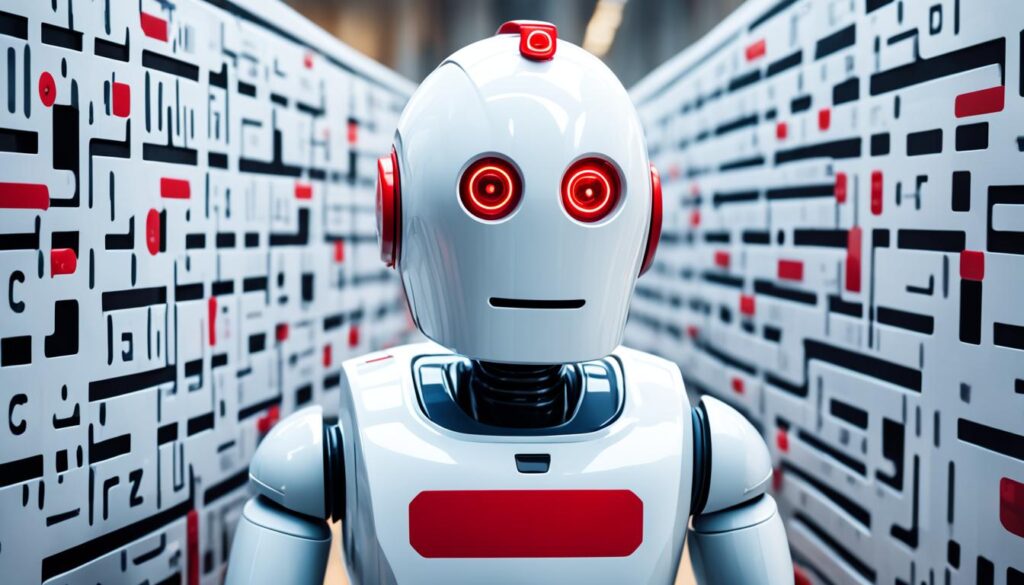
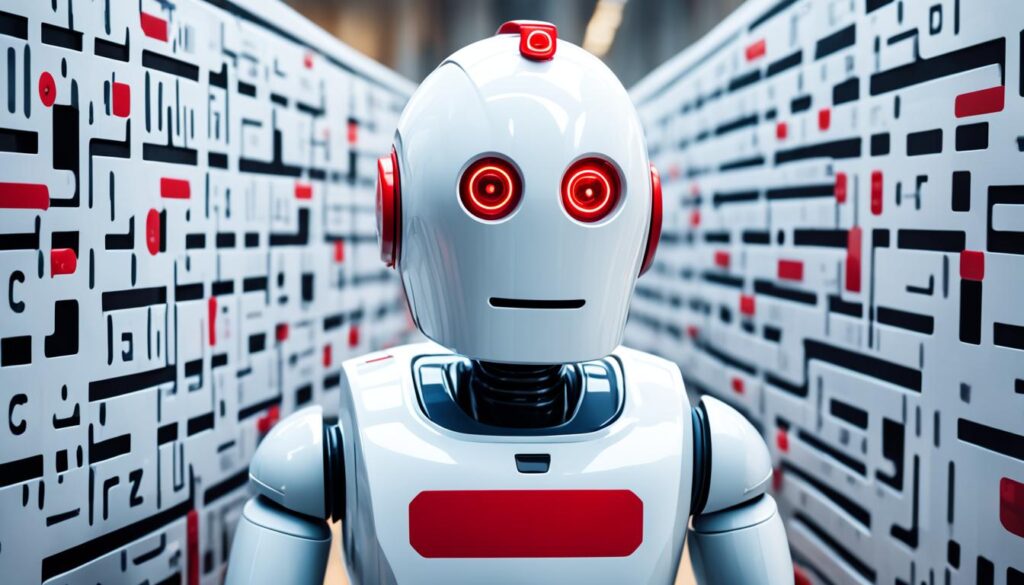
Overall, AI is a valuable tool that can revolutionize customer feedback analysis. However, businesses must navigate the challenges and limitations of AI to ensure accurate, ethical, and impactful analysis that enhances the overall customer experience.
Implementing AI in CRM
Implementing AI in customer relationship management (CRM) is a strategic process that requires careful planning and execution. By harnessing the power of AI, businesses can gain a competitive edge, enhance customer interactions, and make data-driven decisions to drive their success.
One of the key considerations in implementing AI in CRM is ensuring data quality and integration. Clean and accurate data across relevant systems and platforms is essential for effective AI-powered CRM. This involves consolidating and validating data from various sources to create a unified view of the customer.
Choosing the right AI tools and platforms is also crucial. Different AI tools offer specific strengths and weaknesses, so selecting the ones that align with your business goals and CRM requirements is essential. Whether it’s AI-powered chatbots for customer support or predictive analytics tools for sales forecasting, understanding the capabilities of each tool is vital.
Best Recommended and Proven Way to Make Money Online – Click HERE for Instant ACCESS >>
Training and expertise play a crucial role in successfully implementing AI in CRM. It’s essential to have trained professionals who can effectively use AI tools and extract meaningful insights from the data. Ongoing training and development ensure that your team stays up-to-date with the latest advancements in AI technology.
Continuous monitoring and optimization of AI performance are necessary to achieve the desired results. Regularly assessing the effectiveness of AI algorithms and making necessary adjustments will ensure that your CRM system delivers optimal outcomes. This iterative process allows you to refine and improve your AI implementation.
Data privacy and security should be top priorities when implementing AI in CRM. Building customer trust is crucial, and protecting their personal information is paramount. Ensuring compliance with data protection regulations and adopting ethical practices for handling customer data is essential for maintaining a positive reputation and customer trust.
By implementing AI in CRM, businesses can unlock a range of benefits. AI-powered CRM tools can automate time-consuming tasks, enabling your team to focus on more meaningful customer interactions. AI can also provide valuable insights into customer behavior, preferences, and trends, allowing you to tailor your products and services to meet their needs more effectively.
In conclusion, implementing AI in CRM requires strategic planning, data integration, the right AI tools, training and expertise, continuous monitoring, and a focus on data privacy. By embracing AI technologies in your CRM system, you can transform customer interactions, gain a competitive advantage, and make more informed decisions powered by data-driven insights.
Types of AI-Powered Predictive Models
AI-powered predictive models encompass various machine learning algorithms that can be used for customer segmentation and predictive analytics.
Clustering algorithms like K-Means, Hierarchical Clustering, and DBSCAN group similar customers based on their behavior and preferences.
Decision trees, such as CART and Random Forests, split data into branches of decisions to predict outcomes or identify patterns.
Support Vector Machines (SVM) are used for classification tasks and can be applied to customer segmentation.
Neural networks, like Deep Learning and Convolutional Neural Networks (CNNs), are used for complex tasks when large datasets and computational resources are available.
Understanding these different types of AI-powered predictive models helps businesses choose the most suitable approach for customer data analysis needs.
Real-Life Examples of AI in Customer Segmentation
Several companies have successfully implemented AI-driven customer segmentation strategies to enhance their marketing and customer engagement efforts. These real-life examples demonstrate the effectiveness of AI in customer segmentation across various industries. Let’s explore some of these success stories:
Amazon
Amazon utilizes AI to analyze customer data and make personalized product recommendations. By leveraging AI-driven customer segmentation, Amazon can contribute to high conversion rates and customer satisfaction, delivering a tailored shopping experience.
Netflix
Netflix applies AI-driven segmentation to enhance content recommendations and reduce customer churn. Using AI algorithms, Netflix can understand user preferences and provide personalized viewing suggestions, improving customer engagement and retention.
Spotify
Spotify uses AI algorithms to segment users based on their listening habits. This AI-driven customer segmentation allows Spotify to create personalized playlists like “Discover Weekly,” keeping users engaged and satisfied with tailored music recommendations.
Airbnb
Airbnb employs AI to segment its customers and offer personalized travel recommendations. Using AI-driven customer segmentation, Airbnb provides tailored suggestions to different customer segments, enhancing the overall travel experience and increasing customer satisfaction.
Starbucks
Starbucks utilizes AI-driven segmentation to provide personalized promotions and rewards through its app. By analyzing customer data, Starbucks delivers personalized offers to enhance customer loyalty and drive repeat visits.
These real-life examples exemplify the power of AI-driven customer segmentation in improving marketing efforts, increasing customer engagement, and driving business success. By leveraging AI in customer segmentation, companies can better understand their customer’s unique needs and preferences, resulting in a more personalized and targeted approach to marketing and customer interaction.
The Future of AI in Predictive Analytics
In the ever-evolving landscape of data analysis, the future of AI in predictive analytics holds immense potential for advancements and innovation. With the rapid development of machine learning models, such as Transformers, the capabilities to handle and analyze unstructured data will significantly improve. This will enable businesses to extract valuable insights from vast and diverse data sources, empowering them to make informed decisions based on comprehensive analysis.
Explainable AI (XAI) is another promising trend in predictive analytics. As AI-driven predictions become increasingly critical in decision-making, understanding and interpreting the reasoning behind these predictions becomes paramount. XAI techniques aim to provide transparency in AI algorithms, allowing users to comprehend the factors influencing predictive outcomes and build trust in the decision-making process.
Real-time segmentation is poised to revolutionize how businesses respond and adapt to customer behaviors. By leveraging AI-powered tools that can segment customers in real time based on their preferences, behaviors, and interactions, companies can deliver personalized experiences and ensure timely engagement. This dynamic approach to segmentation will enable businesses to stay ahead of customer demands and rapidly adjust their strategies.
The future of AI in predictive analytics also involves generating AI-driven content. With natural language processing and machine learning advancements, businesses can create highly personalized and engaging content for their customers. From automated product descriptions to tailored marketing campaigns, AI-generated content will enable companies to enhance customer experiences and deliver relevant and impactful messaging.
Data privacy and security have become critical concerns in the digital age. As AI plays an integral role in customer data analysis, privacy-preserving techniques will become increasingly important. Businesses must prioritize data protection and implement robust measures to safeguard customer information, ensuring compliance with data privacy regulations and maintaining customer trust.
Edge AI, deploying AI algorithms and models on edge devices, will enable faster and more secure processing. By bringing the power of AI to devices such as smartphones, IoT devices, and edge servers, businesses can analyze data locally, reducing latency and enhancing data privacy. This decentralized approach to AI processing offers significant benefits, particularly in real-time applications that require quick response times.
AI ethics and bias mitigation will continue to be fundamental considerations in the future of predictive analytics. Ensuring fairness in predictions and mitigating biases inherent in AI algorithms will be crucial for building trust and avoiding unintended discriminatory outcomes. To ensure equitable and responsible use, businesses must adopt ethical frameworks and implement clear guidelines when developing and deploying AI systems.
Best Recommended and Proven Way to Make Money Online – Click HERE for Instant ACCESS >>
Integration with the Internet of Things (IoT) will further amplify the capabilities of AI in predictive analytics. By combining AI with the vast network of connected devices, businesses can gain real-time insights into customer interactions, preferences, and needs. This integration will enable companies to proactively address customer demands, optimize operations, and deliver personalized experiences across multiple touchpoints.
Cross-channel segmentation, or the ability to seamlessly segment customers across various channels and touchpoints, will be essential for providing a consistent and coherent customer experience. As customers interact with businesses through multiple platforms, AI-powered tools will enable companies to understand their behavior holistically and tailor experiences accordingly. This comprehensive approach to customer segmentation will enable businesses to maximize engagement and build strong, lasting relationships.
In conclusion, the future of AI in predictive analytics is filled with exciting possibilities. With advancements in machine learning models, explainable AI, real-time segmentation, AI-generated content, privacy-preserving techniques, edge AI, AI ethics, IoT integration, and cross-channel segmentation, businesses will continue to harness the potential of AI to drive innovation, competitiveness, and customer-centricity in customer data analysis. Embracing these trends will empower companies to gain unique insights, deliver personalized experiences, and make data-driven decisions that propel them forward in the digital age.
Conclusion
In conclusion, using AI in customer data analysis has revolutionized how companies analyze and utilize customer feedback. By automating processes and providing accurate insights, AI-powered tools enhance customer experiences by enabling personalized marketing campaigns and improving customer feedback analysis. Successful businesses like Starbucks and Netflix have already implemented AI in customer data analysis, resulting in improved products/services, increased customer loyalty, and higher revenues.
Implementing AI in CRM requires careful planning and consideration of data quality, AI tools, training, and data privacy. However, the benefits are significant. AI in predictive analytics holds great potential for data processing, personalization, and advancements in real-time insights. By embracing AI in customer data analysis, businesses can gain a competitive edge, enhance the customer experience, and make smarter data-driven decisions.
In summary, AI is a game-changer in customer data analysis. Companies can automate processes, gain accurate insights, and improve decision-making by leveraging AI-powered tools. The future of AI in predictive analytics is promising, with advancements in personalization, real-time insights, and data processing. As businesses strive to enhance the customer experience, AI remains a vital tool to drive success and establish a strong competitive position.
Read this article, Analyzing Consumer Behavior: Tools and Techniques for Marketers, for more insights into consumer behavior.